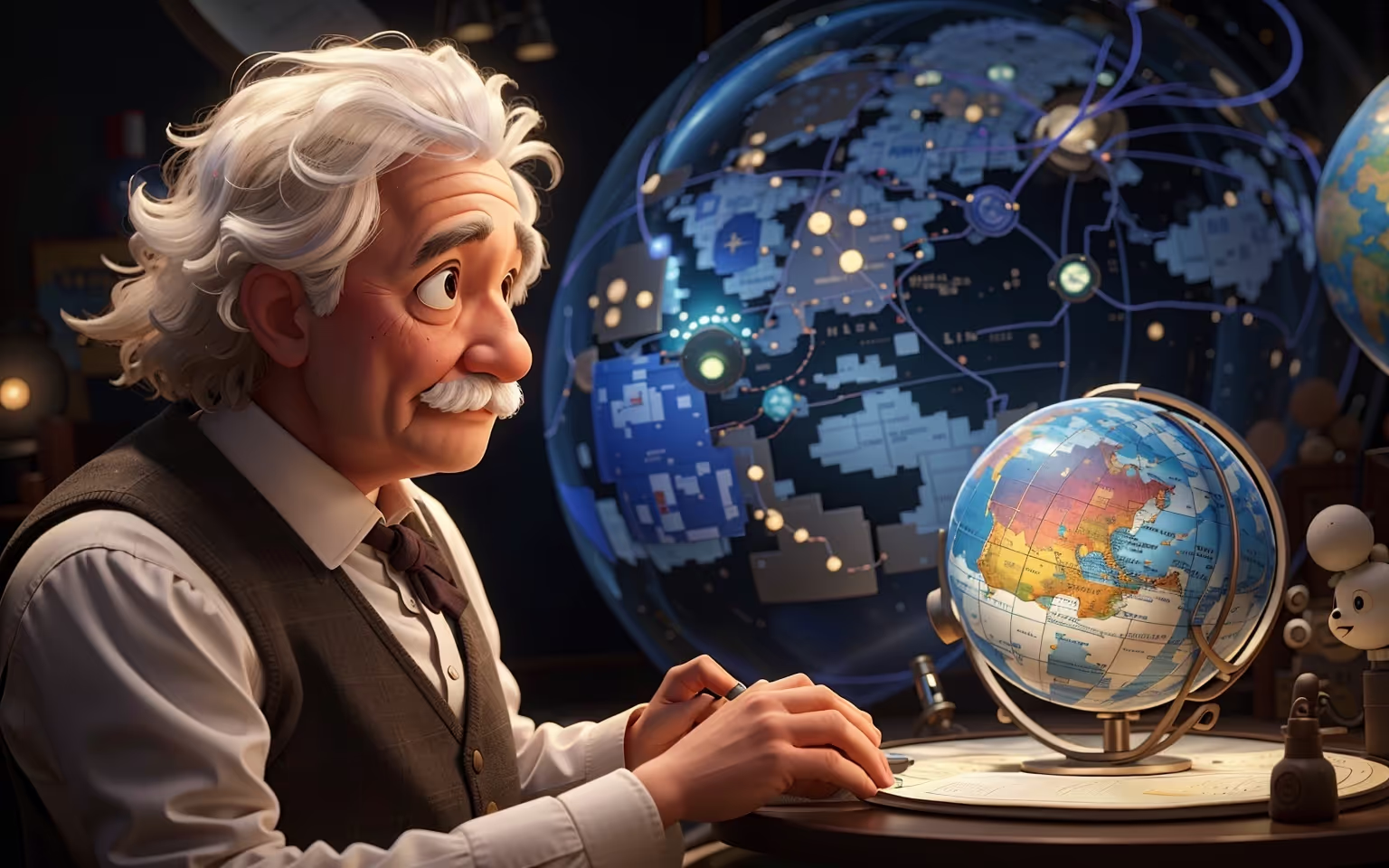
Indistinguishable from Magic: Mapping the Emerging AI Market
“You can't use an old map to explore a new world” – Albert Einstein
Welcome to Part 2 of a 4-part series on the state of AI.
You can revisit Part 1 here for a recap of what has happened during this incredible year of AI.
In Part 2, we walk through the market map of AI and highlight some of the emerging pockets of opportunity.
Coming up, in Part 3 we share an overview of our mental model for assessing AI companies and in Part 4, we profile a number of case studies of how companies are responding to AI from across the Blackbird portfolio.
Part 2: The Emerging AI Market Map
At its most foundational level, the AI market is segmented into the application and “infrastructure stack” layers.
Infrastructure layer companies are building the “pick and shovel” tools for the application layer - the scaffolding required to execute on AI. They are generally API-led targeting developers and ML engineers.
Application layer companies are building AI-enabled apps for consumers and enterprise, either horizontally for role functions or vertically for specific industries.
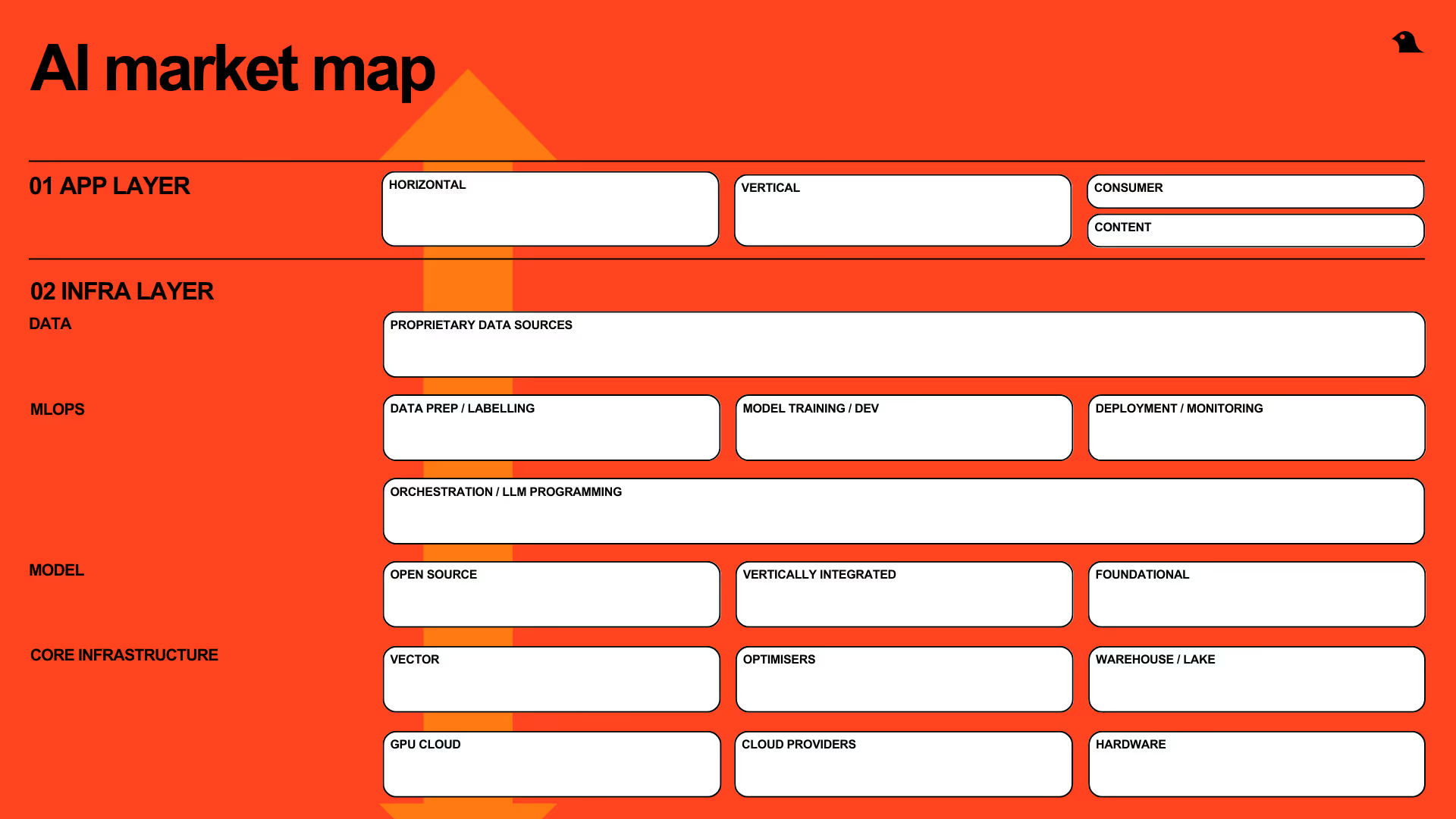
It is interesting to observe how the line between the infrastructure and application layers has rapidly “re-blurred” with AI.
Many years ago, companies used to have to design, provision and host their own infrastructure and this was a source of advantage and differentiation. Over time, infrastructure offerings have shifted to the cloud and strengthened significantly (e.g. “backend-as-a-service”, “platform-as-a-service”, “infrastructure-as-a-service”), meaning application companies could spend more time building products and less time worrying about their backend architecture.
With the latest developments in AI, we are now seeing application companies having to revert and refocus heavily on their infrastructure stack again. The line between being an application and infrastructure company is blurring, again.
Companies from Canva to Salesforce, Intuit, and Intercom are putting more emphasis on their backend stack than ever before, building internal MLOps platforms and teams, re-architecting their data stacks, and training and fine-tuning proprietary models.
The components of the infrastructure stack are once again playing a critical role in product execution and application experience. As a user, you may not notice a meaningful change if Canva switched cloud providers, yet every new underlying LLM release comes with a major jolt to the UX of Canva Magic.
Correspondingly, infrastructure companies are moving into the application layer.
OpenAI started life as a pure-play infrastructure company, GPT-3 was only available as an API for developers to integrate into their own applications. It then launched ChatGPT as a consumer application in November 2022, e-commerce plugins in March 2023, and ChatGPT Enterprise in August 2023, reinventing itself as a hybrid infra-application company. OpenAI is on track to hit $1B revenue this year.
The other line that is heavily blurring is that between consumer and enterprise at the application level.
We have seen this trend for years with the emergence of “product-led growth”, “bottom-up adoption”, and the “prosumer” category. But, we have not seen applications with such clear value for both consumer and enterprise use cases, driving near universal adoption from Day 1.
Infrastructure stack opportunity
There are 4 primary layers within the infrastructure stack, bottom-up: core infrastructure, model, MLOps, and data.
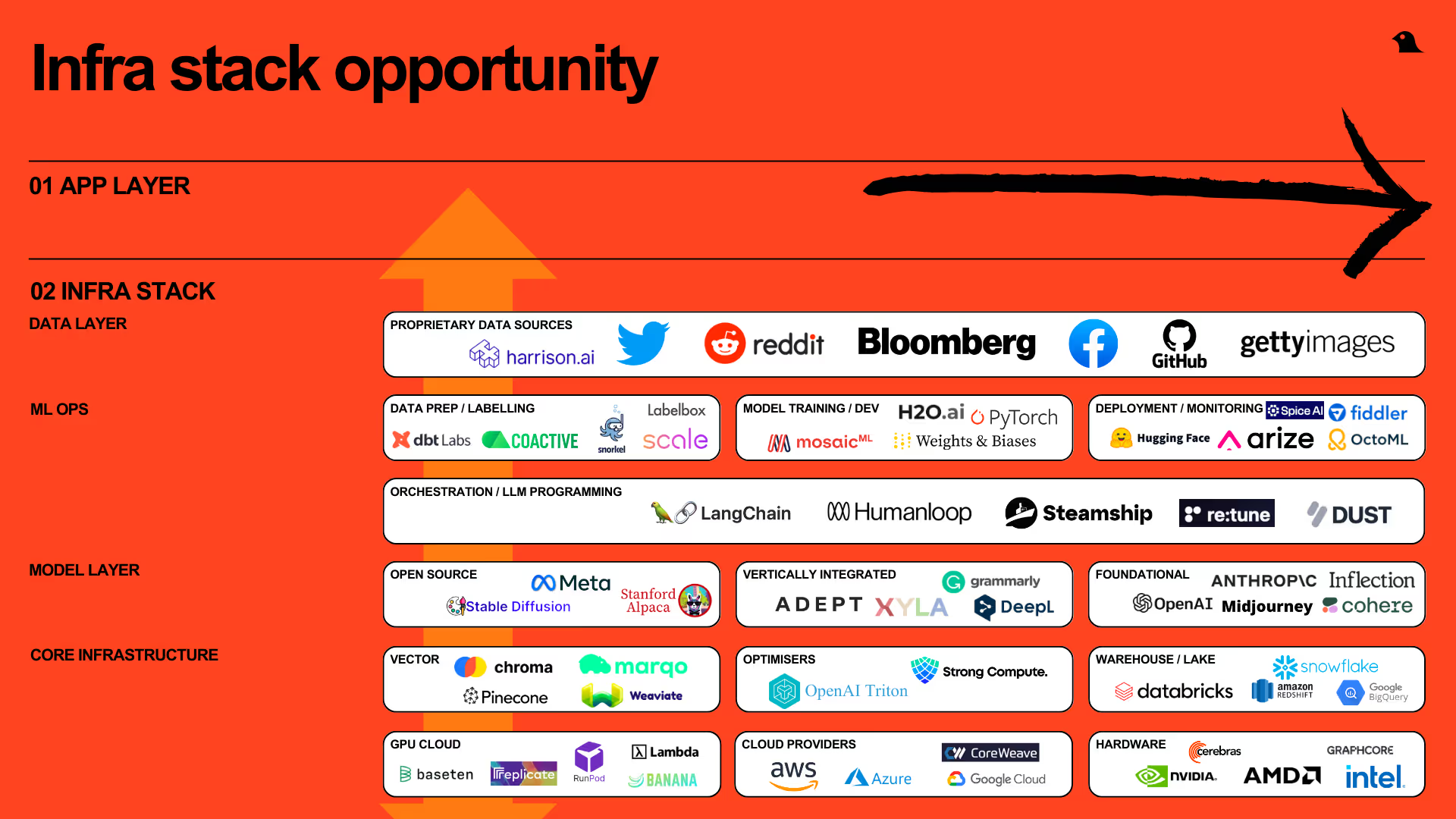
Core infrastructure layer
You may think that core infrastructure such chips and hardware (what a year for Nvidia!), data warehouses, data lakes (Databricks, Snowflake), and cloud servers (AWS, Azure, Google Cloud Platform) would be well accounted for.
But, there are many pockets of opportunity, many new and emerging, that excite us.
One area is cloud optimisation. In 2022, we co-led the seed round into Stong Compute which optimises the core infrastructure for model training. Training is costly, time-consuming and painstakingly manual, and Strong Compute drives a 10-1000x improvement in training speeds and significant cost savings.
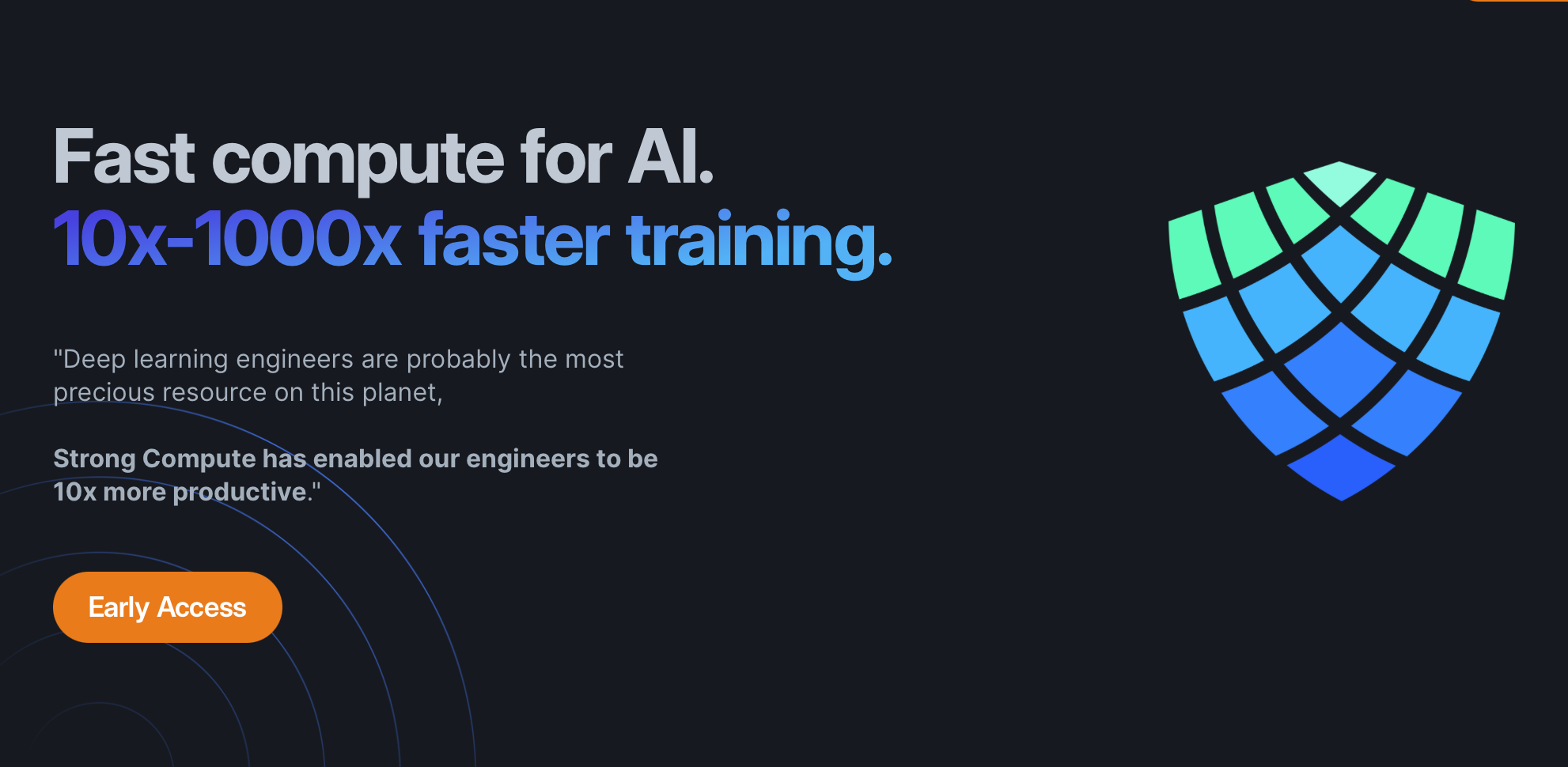
Vector databases are another category, garnering attention because of the integral role they play in tandem with LLMs.
Commonly, developers want to point a LLM at specific datasets, like an enterprise’s proprietary data or knowledge base, to generate responses that are more contextualised. These datasets are typically large, complex, with different structures and tile types, which is where vectors step in. Vectors can filter through multi-modal data repositories to retrieve the most relevant data at speed.
Operating tangentially to vector databases is Marqo, which we recently invested in. Marqo is building an open source, end-to-end, AI-search solution on top of vector-based capabilities and is driving meaningful outcomes for customers with real-time search across complex data sets.
You can read our investment notes on Marqo here.
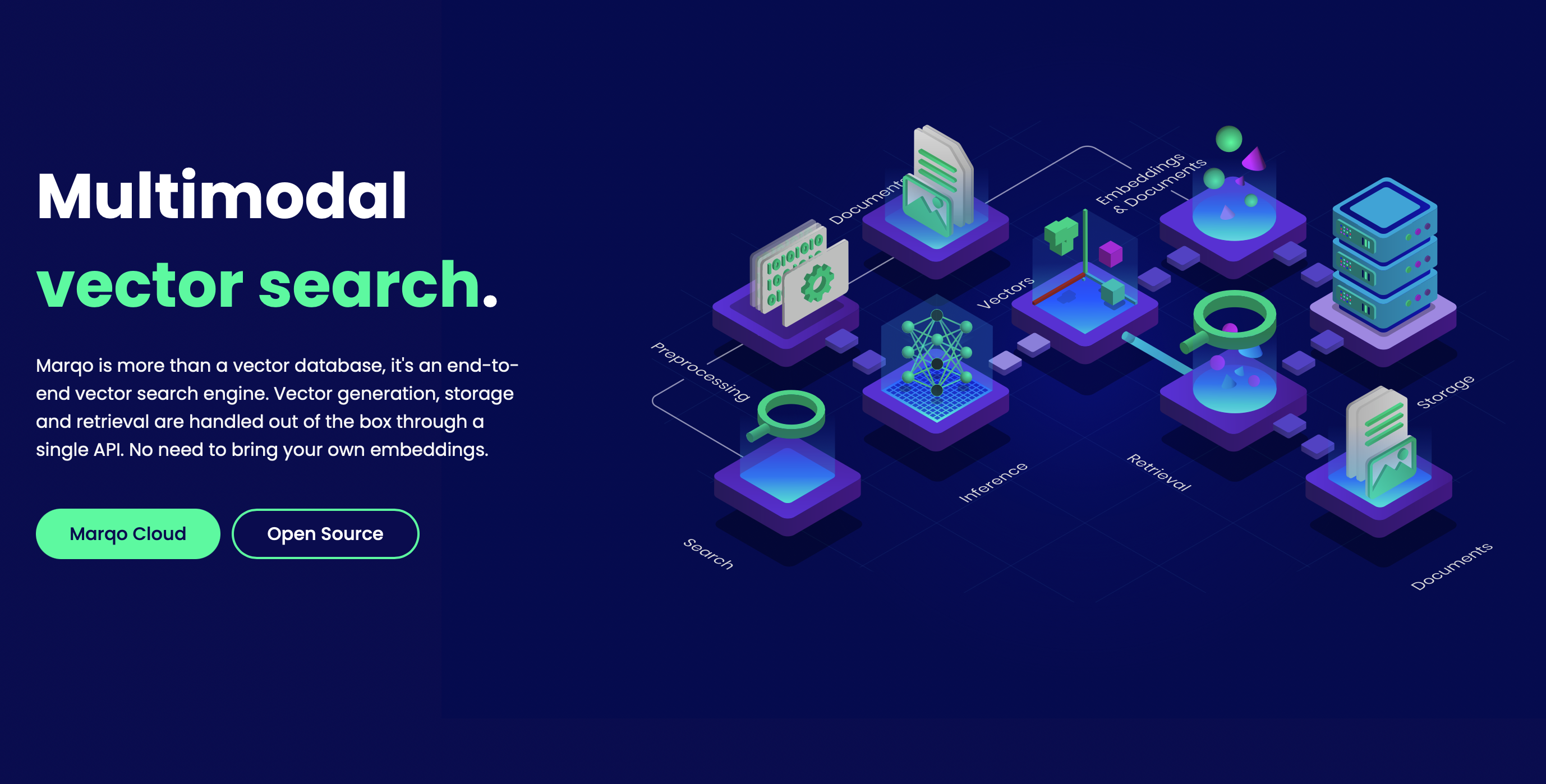
Model layer
Foundational LLMs - GPT-4 (OpenAI), Claude (Anthropic), Pi (Inflection), Bard (Google), and Midjourney - have been getting the majority of attention in the infrastructure stack.
These businesses have raised vast amounts of capital, operate on big budgets (GPT-4 cost $100M to train), and are achieving levels of performance for generalised purposes that will be hard to chase down from a cold start.
One area of interesting opportunity is in the vertically-integrated LLM category. For example, specialised models are being trained for niche use cases in biomedicine (Xyla, Inceptive), coding (Codex), character chatbots (Character AI), and language translation (DeepL).
The verticalised-model opportunity is heightened by “complexity”. Complexity creates room for the performance of general foundational models to be improved upon, generally created by:
- Regulation e.g. HIPAA for healthcare increases compliance requirements.
- Need for accuracy e.g. obvious risks in healthcare but also in chemistry and law with consequences already making headlines.
- Niche industry data and syntax e.g. Codex, a code-specific model trained on a large, custom dataset (54M GitHub code repositories) and on the syntax of coding languages.
But, GPT-4 is impressively performant, and so the open question remains: in which domains will specialised models outperform the constantly improving foundational models?
The open source vs. closed source debate is also interesting to explore. Open source models are currently still inferior, but are rapidly improving and harnessing community support.
One question we’ve stewed on is what is Meta’s skin in this game. Why is Meta i developing their own open source models like Llama? It’s hard to buy into “open sourcing makes LLMs safer and better by inviting outside scrutiny”.
We think it is likely that Meta sees LLMs as a threat to their ad-driven revenue model, since LLMs play a critical role in the content and creative process, eating into marketing spend. With Llama, Meta is commoditising its complement.
MLOps layer
The data preparation and labelling category is experiencing a reshuffle.
On one hand, historically human-enabled data labelling and tagging workflows can be better automated and are getting disrupted. On the other, LLMs and AI applications are hungry for data, and there are big gaps in data cleaning, structuring and preparedness: point a LLM at file types such as .pwt, .xlsx, .pptx and performance can wane quickly. This is creating opportunities - Unstructured is one company attempting to fill in the gaps and we expect others to follow.
In model deployment, we have invested in Spice AI which offers developers an “AI backend-as-a-service” with a primary focus on time series datasets.
If Databricks, Hugging Face, and GitHub had a baby, Spice would be it! Spice marries an enterprise-scale platform with the building blocks developers need to spin up AI-intelligent applications – think, petabyte scale real-time and historical data capabilities, ML training pipelines, function compute, hosted models and database storage – with a library of public and private time series data sets to plug into models.
To date, Spice’s focus has been on Web3 use cases, given the ubiquitous importance of robust real-time blockchain data for most companies in the space. Read our investment notes on Spice AI here.
Data Layer
We expect new “data-as-a-service” and “model-as-a-service” business models to become prevalent.
Many of today’s largest companies were built on the business of selling data - Facebook, Google, Oracle. But this has been predominantly based on the sale of personal data for targeted marketing. Selling large, generalised, unstructured human-generated datasets for self-supervised model training is a completely new ball game.
Swift moves from the likes of Reddit and Twitter, which sit on unique and vast conversational datasets, to restrict API access to their platforms is a signal of the opportunity.
Bloomberg recently launched BloombergGPT, a specialised LLM trained on its proprietary financial data. The outcome of copyright cases such as Sarah Silverman vs. OpenAI and Getty Images vs. Stable Diffusion will have a significant impact on this category, and set the tone for how organisations can control and protect the use of their data for model training.
Application Layer Opportunity
With powerful machine learning capabilities now a simple API away, there has been an explosion of activity at the application layer.
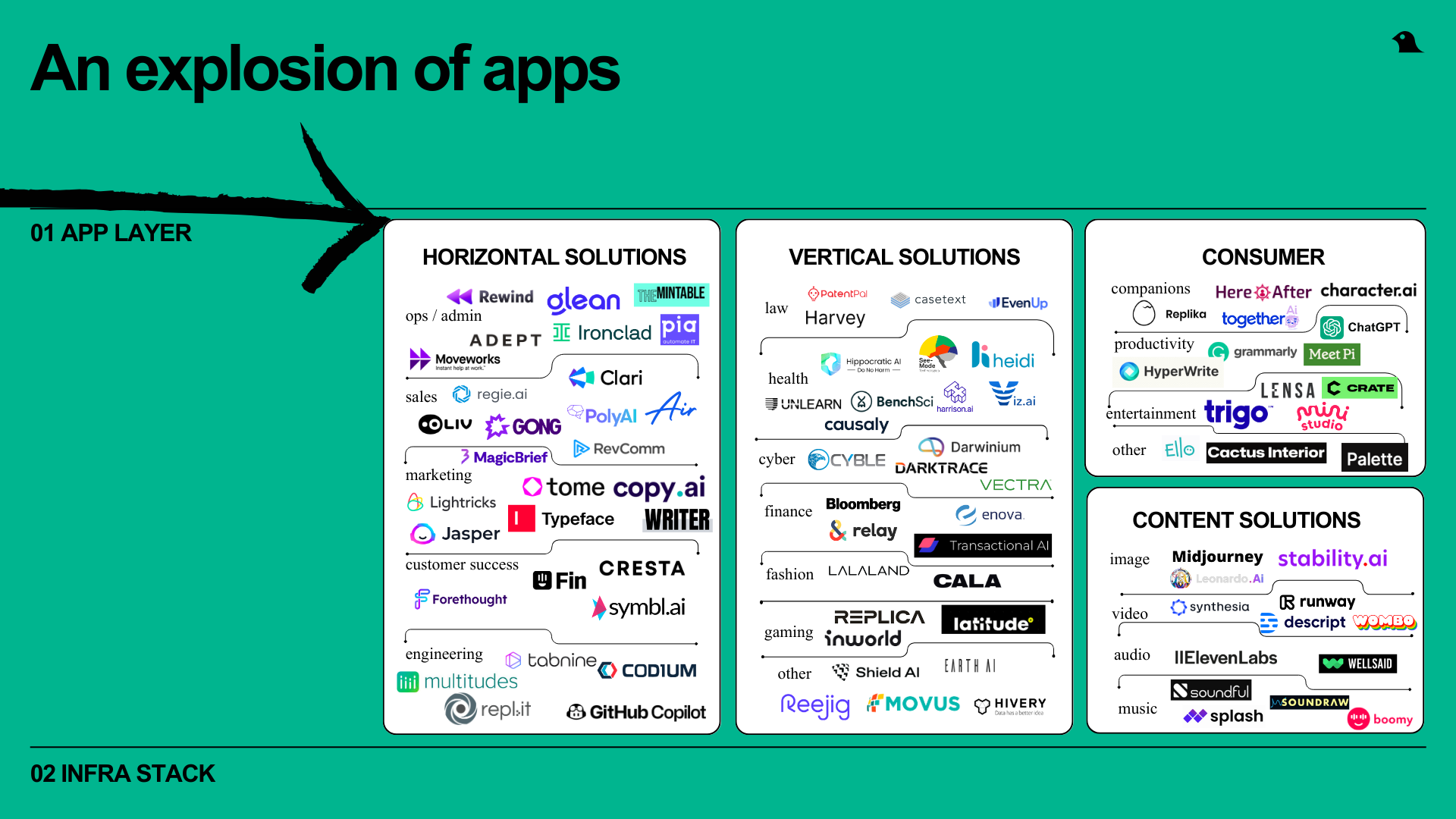
For enterprise, we are seeing activity across both the horizontal and vertical stacks.
In the horizontal category, we have invested in a number of AI-core companies including The Mintable (for people managers), MagicBrief (for marketers), and Multitudes (for engineers) and are seeing many interesting opportunities.
We have also invested in industry-focused applications such as healthcare (Harrison AI, See Mode, Heidi), cyber (Cyble, Darwinium), finance (Transactional AI), industrials (Movus, Earth AI), and retail (Hivery).
Within consumer, an interesting category to emerge is a new genre of companion applications, buoyed in large part by the mental health and loneliness epidemics. Influencer Caryn Marjorie launched a “virtual girlfriend” chatbot priced at $1 per minute and made $72,000 in the first week. Replika, a leading companion player, had to rapidly reverse a decision to put a filter on erotic chat on the platform after an en mass revolt from users.
This year to date, we have welcomed 5 additional application companies to the portfolio that we are excited to announce in the near future.
______
Coming next
The AI stack is rich and growing and we are excited to see how it continues to unfold.
We love meeting with founders no matter where in the stack they are building - and please reach out to Silk on LinkedIn / X and Tom on LinkedIn / X.
Coming up next is Part 3, where we share more on our mental model for approaching AI companies and Part 4, where we explore case studies across the portfolio.
A special thanks to Luke Kim, Alex Edwards, and Jesse Clarke for their help in bringing this piece to life.